From AI POCs to MVPs: A proven process for speed & success
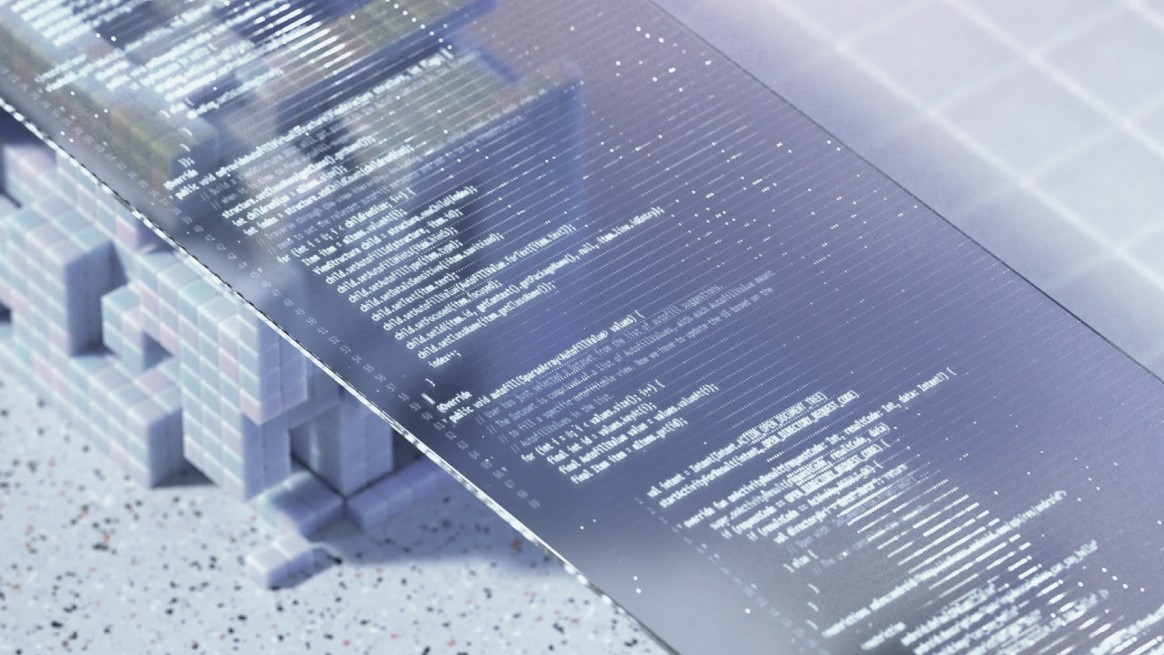
In January 2025, about 90% of companies admitted struggling to execute or scale their AI initiatives.
Some of this is out of your hands (e.g., GPU shortages), but a lot of the roadblocks come from within: slow-moving processes, resistance to change, and teams working in silos. These challenges can stall AI projects before they even get off the ground.
At DEPT®, we’ve spent the past decade helping companies cut through the noise and turn AI ambitions into real-world results. Our AI accelerator program speeds up prototyping and scaling, tapping into a library of pre-built models to get concepts off the ground fast. With deep expertise in data, engineering, innovation, and product development, we’ve helped many global brands successfully launch AI POCs. This hands-on experience allows us to bridge the gap between ideation and execution—ensuring AI solutions drive measurable business impact, not just hype.
Here’s our proven method to deliver MVPs on time and budget.
Key process principles
When it comes to AI, speed matters—but so does structure. The right balance means that AI solutions move from idea to execution without getting stuck in endless iterations. Here’s how to get it right:
- Rapid delivery: Work in short, iterative cycles to develop AI POCs quickly. The goal is to show business impact fast, not get bogged down in perfectionism.
- Measure success: Define clear, quantifiable success criteria upfront. Growth is great, but AI can also reduce churn, improve efficiency, and enhance quality—so focus on what moves the needle for your business.
- Enablement: Don’t reinvent the wheel. Leverage AI accelerators and existing implementations to fast-track development so you’re building on a foundation rather than starting from scratch.
A successful AI POC-to-MVP follows a three-phase cycle:
- Ideation phase: Identify challenges, refine opportunities, and prep for POC development
- POC realization: Build and test a functional AI prototype
- MVP spin-off: Transition the validated concept into a Minimum Viable Product (MVP) for broader adoption
Ideation phase
The first step is determining if AI can make an impact and whether it’s feasible to implement.
This isn’t a solo effort. It takes a well-rounded team to spot opportunities, troubleshoot challenges, and realize success. A data & AI strategist, digital strategist, channel specialist, enterprise architect, project manager, and UX/UI designer all bring unique perspectives to the table. Together, they shape the vision, validate the approach, and set the foundation for an AI solution that delivers real results.
- Pain points: Always start with your business struggles before considering whether AI could be a solution.
- State of AI: Align stakeholders with the latest AI advancements and their business potential. AI is constantly evolving! We renew our “State of AI” every few months.
- Opportunity mapping: In collaboration with your team, map out potential AI opportunities. Focus on three key areas: process efficiency, delivery efficiency, and commercial growth opportunities.
- Technical feasibility assessment: Perform a high-level assessment of each AI idea’s feasibility based on current capabilities, data availability, technical complexity, and internal processes. Determine if there are significant obstacles or dependencies.
- Prioritization with RICE Scoring: Rank AI ideas based on Reach, Impact, Confidence, and Effort. Prioritizing AI initiatives through this framework ensures your resources are allocated to AI projects with the highest potential business value. That way, you can drive measurable improvements in efficiency, customer experience, or revenue.
- POC Sign-off: Select top opportunities and lock in the scope for the next phase.
POC realization
Now it’s time to build.
The goal is function over flash–an AI POC that aligns with your business objectives. The team involved in this phase typically includes key roles such as channel specialist, AI engineer, prompt engineer, full-stack engineer, UI designer, and project manager.
- Implementation planning: Target the delivery of 2–3 AI POCs within your timeframe.
- Define success metrics: Establish business KPIs that will measure POC impact.
- Workflow & UX design: Map AI workflows and create a user-friendly prototype.
- AI & front-end engineering: Develop and integrate the AI model into a working interface. This includes selecting the appropriate AI model architecture, training it with relevant datasets, and fine-tuning it to ensure optimal performance. On the front-end side, the AI model is incorporated into an intuitive user interface, allowing seamless interaction with end users.
- Pilot release & feedback loop: Deploy to a test group (real users), gather insights, and iterate quickly. Your AI and prompt engineers team should continuously optimize the POC based on user feedback. Implement updates and new releases daily to test and refine improvements rapidly.
- Conduct a comprehensive review: Analyze pilot data, user feedback, and performance metrics against the agreed POC success criteria.
MVP implementation
If your POC succeeds, it’s time to move toward an MVP. This is where things get real—you’re no longer proving a concept. You’re setting the foundation for a full-scale AI deployment.
To do this smoothly, you’ll need a DevOps specialist on board. They’ll help streamline CI/CD pipelines, automate deployment processes, and ensure your infrastructure is built to scale. Plus, they’ll integrate monitoring solutions to keep your AI model running optimally in production—so it performs just as well in the real world.
- Team alignment: Establish a joint project team, including AI engineers, business leads, and UX specialists.
- Development-ready design: Finalize technical specs and implementation roadmaps. Designers should concentrate on creating development-ready designs with full annotations, including acceptance criteria.
- Integration planning: Align AI models with existing business systems and data pipelines.
- MLOps implementation: Set up machine learning pipelines for automation and continuous model updates.
- MVP release: Deploy to an extended user group for real-world testing and iteration. After launching the MVP Pilot, it’s essential to remain available for user feedback and to optimize the product weekly. Sharing release notes regularly helps drive adoption and keeps users engaged.
Organizational culture: The missing piece
Even the best AI solutions fail without buy-in.
Getting AI to stick isn’t just about the tech—it’s about the people using it. Keeping teams in the loop on AI advancements and how they impact daily work is key to making adoption smooth. The best way to make that happen? Identify AI champions within your team who can advocate for the change and share knowledge across departments. Launch personalized AI learning tracks to help employees feel confident and ready to work with AI-driven tools.
The real magic happens when AI is embedded seamlessly into workflows, with clear success metrics and rapid iteration. Start small, move fast, and build momentum—because AI only works when your people do too.