Stop overvaluing your channels – use a data driven attribution model
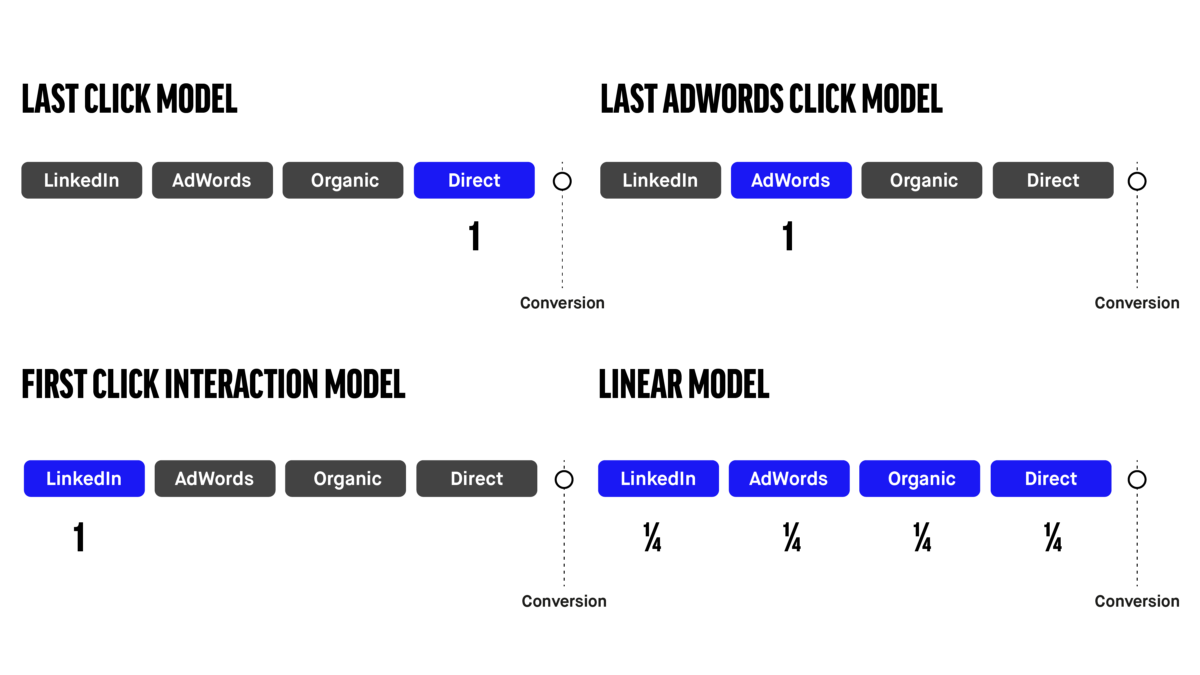
Instead we should use a data driven attribution model
This flaw can be avoided by blending the power of business knowledge and data to build a data driven attribution model. Such a model incorporates all possible combinations of channels in a customer journey and evaluates their conversion rate, which is obtained from historical data. This information is used to algorithmically determine the added value of a specific channel at a specific point in the customer journey. A popular algorithm to conduct these kind of calculations is the Shapley Value from the Cooperative Game theory, which I will not discuss in detail because it is clearly described in this paper and more general by Google here.
The limitations of Google
Not surprisingly that Google incorporated a data driven attribution model in their Google 360 suite. Although this is a major leap forward in correctly attributing channels using Google Analytics, in my opinion the data driven attribution model provided by Google has several strong limitations. Below I briefly touch upon the major limitations:
- Default Channel Grouping Only
- Maximum Customer Journey Length
- Predetermined Business Rules
- No omni-channel Attribution Supported
1. Default channel grouping only
Firstly, Google’s data driven attribution model can only calculate the attribution values over the default channel grouping (see link). Meaning that if you want to get both aggregated and detailed insights into the channel performance, you need to adjust your default channel grouping in a separate view to get a different perspective. Besides the fact that this approach could solve the limitation, it is very prone to errors and not user friendly at all.
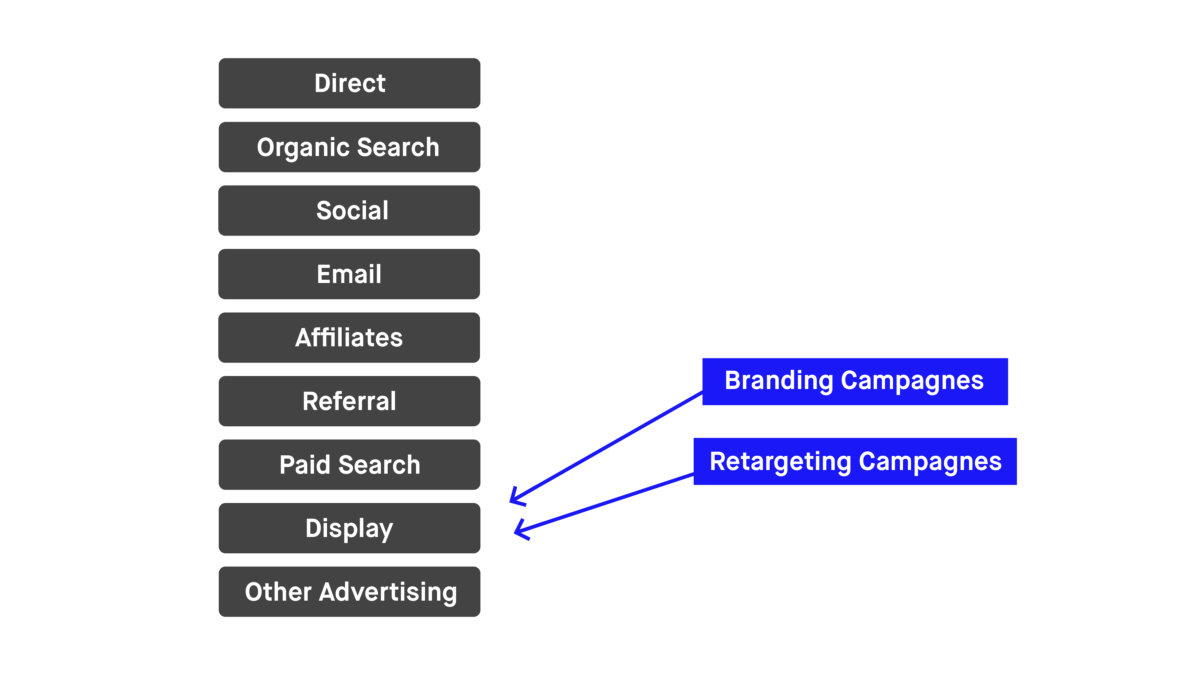
2. Maximum customer journey length
Secondly, the model can only include customer journeys containing up to four touchpoints (link), meaning that customer journeys that may have taken more than four touchpoints are trimmed back to the last four (see image below). This is a major limitation for businesses that sell products that typically require more than four interactions due to, for example, a lengthy orientation process.
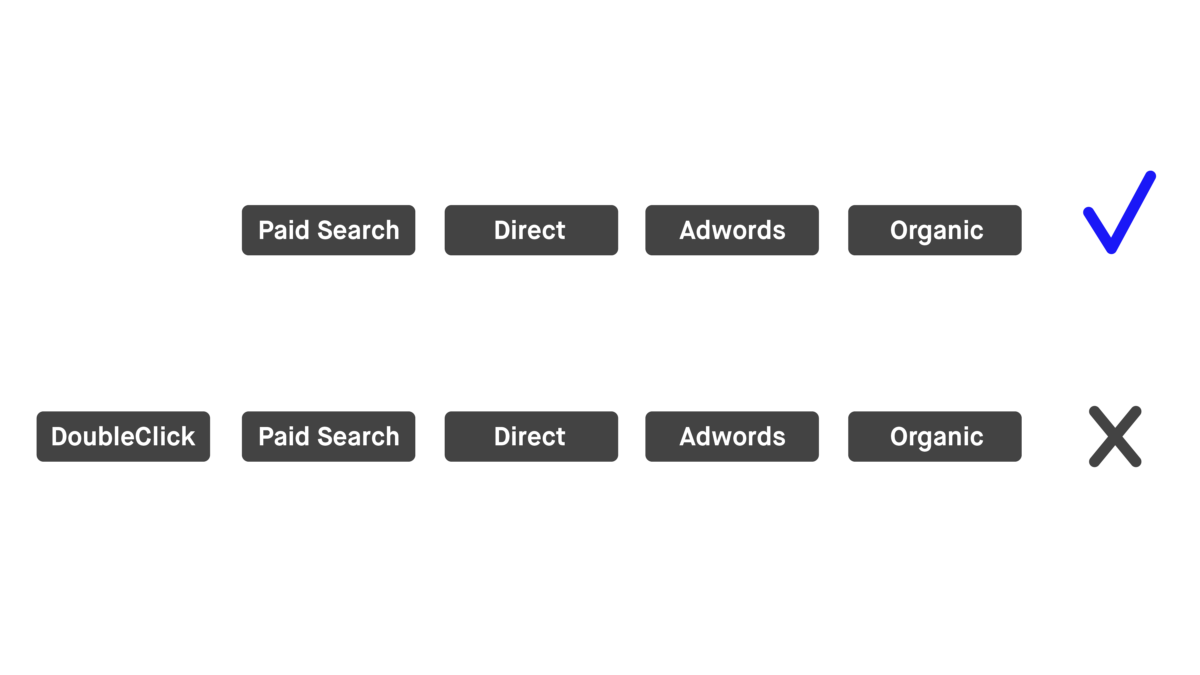
3. Predetermined business rules
Additionally, for some industries, it is common that there are many website visits required to gain the trust of a new customer before the first purchase is conducted. But when this tipping point is reached, each consecutive visit results in a purchase, which should be attributed among the channels in the orientation process as well. To summarize, most businesses and industries need to define their customer journeys with great care to effectively and efficiently use a data driven model to correctly determine channel performance.
Thirdly, the business rules applied to the data by Google are unknown and therefore cannot be customised to adhere to a specific business or industry characteristic. In more detail, the definition of a customer journey is crucial for a data-driven attribution model and is often industry and sometimes even business depended. For instance, touchpoints shortly after purchase could be considered as being part of the previous customer journey’s loyalty loop (link) instead of being the start of a new customer journey of that customer.
4. No omni-channel attribution
Lastly and most importantly, omni-channel attribution is not possible with the data driven attribution model provided by Google because the customer journeys are constructed based on data collected by Google products. In other words, it is desirable to incorporate touchpoints from non-Google data sources (CRM, Email, in-store, etc) into the customer journey’s before using the data driven attribution model. Unfortunately, Google’s model cannot be used with external data sources and thus cannot be applied on datasets that contain a richer more complete picture of the omni-channel touchpoints of the customer journey’s.
Solution: custom data driven attribution model
Considering the limitations of Google’s data driven attribution model, I decided to develop a data driven attribution model that is not subject to the above-mentioned limitations and can be used with a basic Google Analytics accounts as well. In more detail, the Shapley value Cooperative Game algorithm is used in my custom data driven attribution model, which I developed using the open-source software R (See link). By combining the flexibility of R, over 1000 lines of manually written code, and raw data of the business, it is possible to create a custom data driven attribution model that can be shaped and tuned to the needs of an individual business. In this section I briefly elaborate how this custom model avoids making the same mistakes as Google’s data driven attribution:
- Custom Channel Grouping Supported
- Lengthy Customer Journeys Supported
- Custom Business Rules Supported
- Omni-channel Attribution is possible
1. Custom channel grouping supported
The first major advantage is the ability to define multiple sets of channel groupings, which are used to algorithmically calculate channel performance. In other words, this flexibility provides the ability to easily zoom in to specific channel groupings to evaluate the performance in more detail.
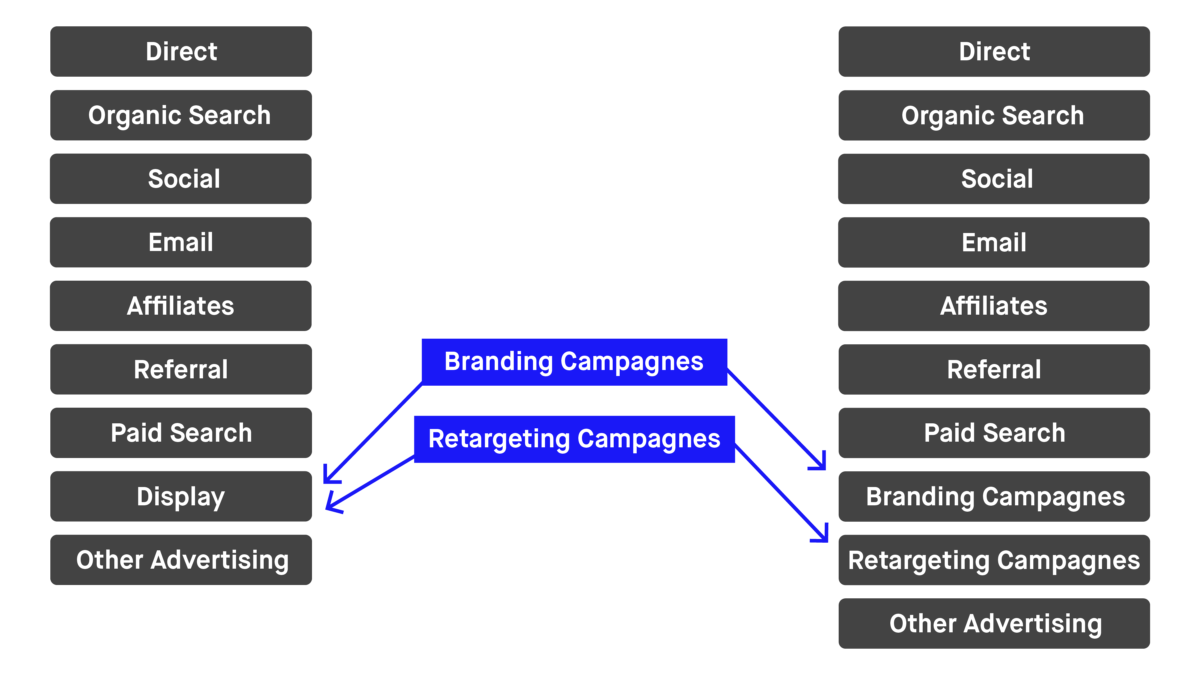
2. Lengthy customer journeys supported
The second major advantage is the possibility to customise the length of the customer journey over which the attribution model calculates the channel performance. Due to this feature, businesses with products that typically have a long customer journey can also apply this custom data driven attribution model without excluding many crucial upper funnel touchpoints.
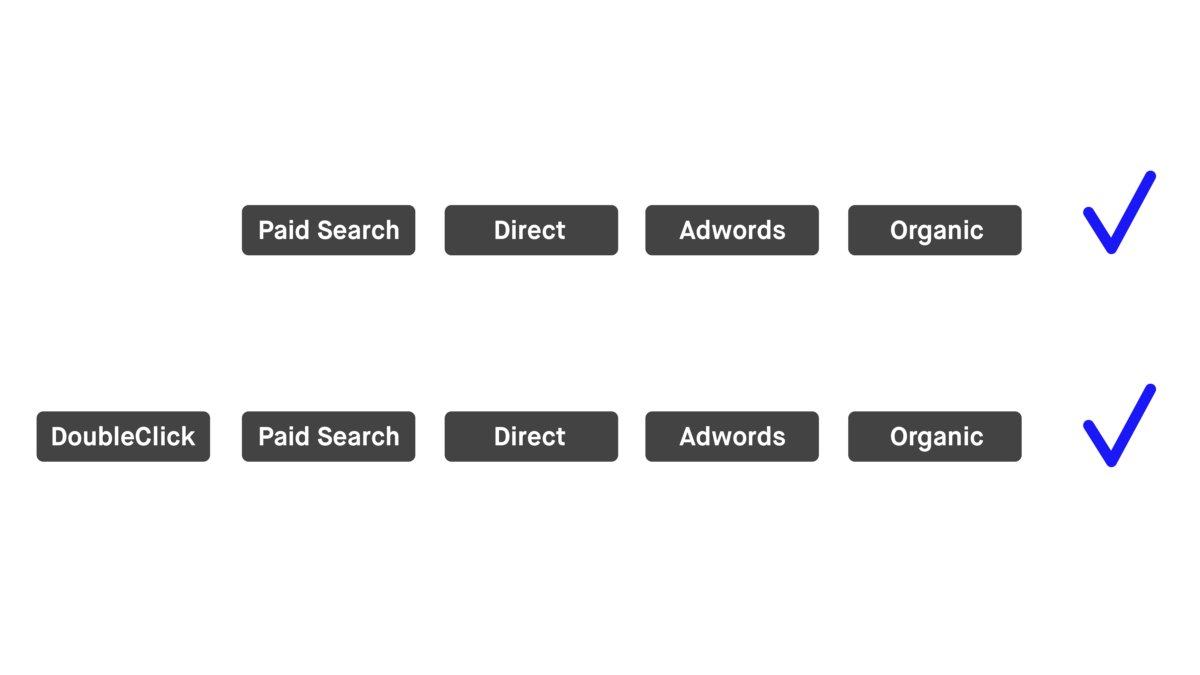
3. Custom business rules
Furthermore, one of the strongest limitations of Google’s model is all business rules about customer journeys are defined for you and cannot be customised or adjusted. This may have a tremendous impact on the outcome of the model and therefore cannot and should not be standardized.
In relation to that, the custom data driven attribution model works with the raw data and therefore enables the user to clearly define the business rules applicable to the customer. This means that the customer journeys can be customised to incorporate business and industry specific rules (see section ‘Predetermined Business Rules’). Furthermore, this does not only make the attribution model applicable to all businesses across different industries, but also makes the attribution process completely transparent since all details about how the attribution is conducted are available and can be explained in detail.
4. Omni-channel attribution is possible
The custom data driven attribution model is not subject to the Google environment and can be used with external data sources (CRM, Email, instore, etc), which enables omni-channel attribution. In other words, when a Data Management Platform (DMP) is in place, which links user ID’s from different data sources together, the custom data driven attribution model can be used to algorithmically calculate omni-channel performance.
But wait, there is more
Besides solving the limitations of Google’s data driven attribution model, I was convinced that there was more to gain and came up with some extensions to further leverage the output of the data driven attribution model. Some opportunities I am thinking of are extensions like a “Return on Ad Spend (ROAS) analysis tool” and a “Channel weight dashboard”.
The former provides insights about the return on ad spend from each customised channel group based on the data driven attribution value and the budget invested in that channel. This provides the opportunity to better determine how much each channel generates in revenue considering the budget invested. The latter provides valuable insights about the performance of each channel for each step in the customer journey. This can be used to determine in which phase of the journey a channel performs best. For instance, it is most likely that some channels are more effective in prospecting while others are more effective in generating conversions.
Recap
To conclude, data driven attribution is a non-arbitrary and correct form of determining channel performance and must be incorporated by all companies that are interested in the effect and results of their marketing budgets. Furthermore, using raw data enables us to fully customize the data driven attribution model in terms of business rules applied, the length of the customer journeys, and the detail of channel grouping used by the Shapley algorithm.
Additionally, it will be crucial that data driven attribution models are flexible and able to be used in combination with different data sources. Predominantly because customer journeys will increase in complexity and the boundary between online and offline experience will blur, which requires an omni-channel attribution approach. Besides that, we all should first interchange our current attribution models for a data driven attribution model because it simply provides the true non-arbitrary performance per channel and shields us from overvaluing or undervaluing our channels.
More Insights?
View all InsightsQuestions?
Director, Data & AI