The Trade Desk’s new audience predictor: Early performance findings
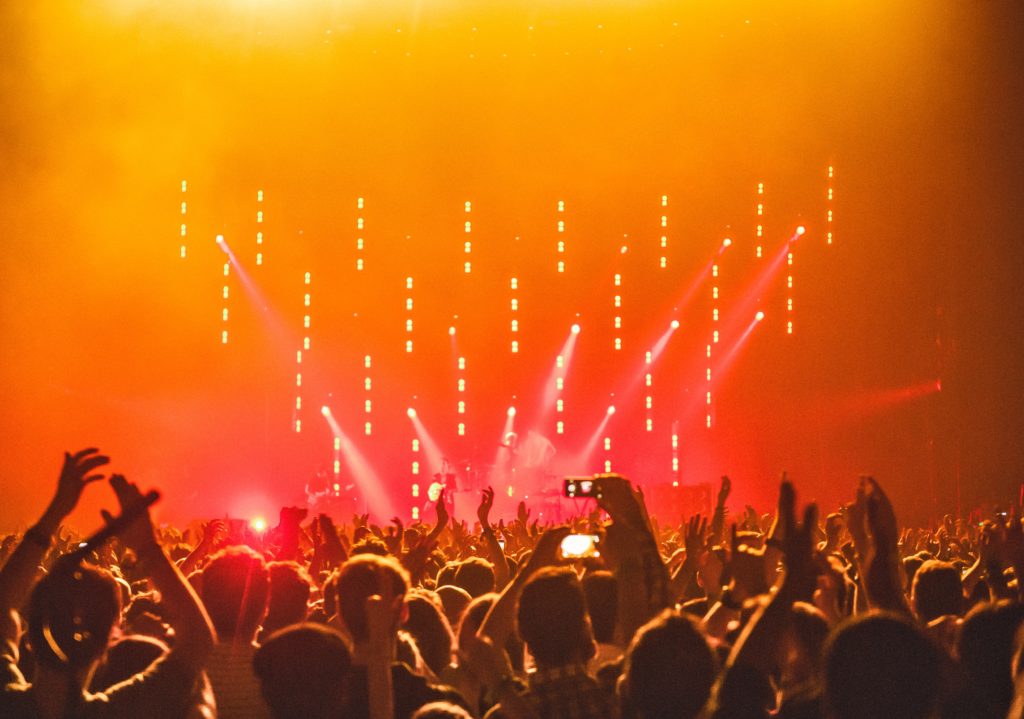
Recently, our preferred DSP (Demand Side Platform), The Trade Desk (TTD), upgraded a targeting LaL (lookalike) feature that we previously tested with decidedly mixed results for clients. So as you can imagine, we were excited to test out these new enhancements as TTD offered the potential to scale our buys with precision, accuracy, and better performance.
The featured product in question is TTD’s Audience Predictor. TTD builds its LaL by indexing known converters against 3rd-party data and then seeing which 3rd-party data segments over-index. The engineering team at TTD really nailed this one by upgrading the following:
- Better matching: You can now create LaL models that closely resemble converters by including the highest-value segments while excluding the lowest-performing segments.
- Improved sample rates: Instead of looking at 1 in 1000 converters to find attributes to build a LAL off of, TTD makes it possible to look at 1 in 10 converters, allowing us to sample a larger scope of people
- Faster audience creation: The system looks for new audiences every 5 minutes.
- Better optimization: The system optimizes automatically toward top-performing segments.
I was lucky enough to test the new Audience Predictor on a client and have seen some really promising results so far. Here’s a summary of the test:
Client: A cloud-based software company looking to attain aggressive growth goals for paying users, while maintaining CPA goals.
Challenge: The previous targeting practices, such as reaching users by job functions that typically convert, had limited scale and weren’t converting at the rates the client wanted.
Solution: We began looking for more creative ways to build custom audiences and decided to tap into the wealth of 1st-party data within TTD by indexing converted users against 3rd-party data to build a LAL audience. Working within the DSP gave us full transparency into which data elements were and weren’t included in the LAL, as well as which elements contributed most to performance.
Results: By targeting based on behaviors rather than job function, we were able to greatly expand the universe of who was being targeted, and meet our client’s CPA goals. Although only 8% of the total spend was allocated to this test, 13% of conversions were generated. With these results we can confidently ramp up spend.
Our CPA dropped 52% from April to May while spend doubled during that time. From May to June, our CPA continued to drop with more budget allocated MoM.
With our client’s long sales cycle and seasonal fluctuations, it’s too soon to analyze the real success of this test. But I’m impressed so far and look forward to continuing testing this functionality.
Ready for better audience targeting? The DEPT® team can help! Reach out to us with any questions.
Learn more about our data and intelligence offering
Explore
Questions?