Mastering the future of Amazon Ads: Purpose-driven measurement and machine learning AI
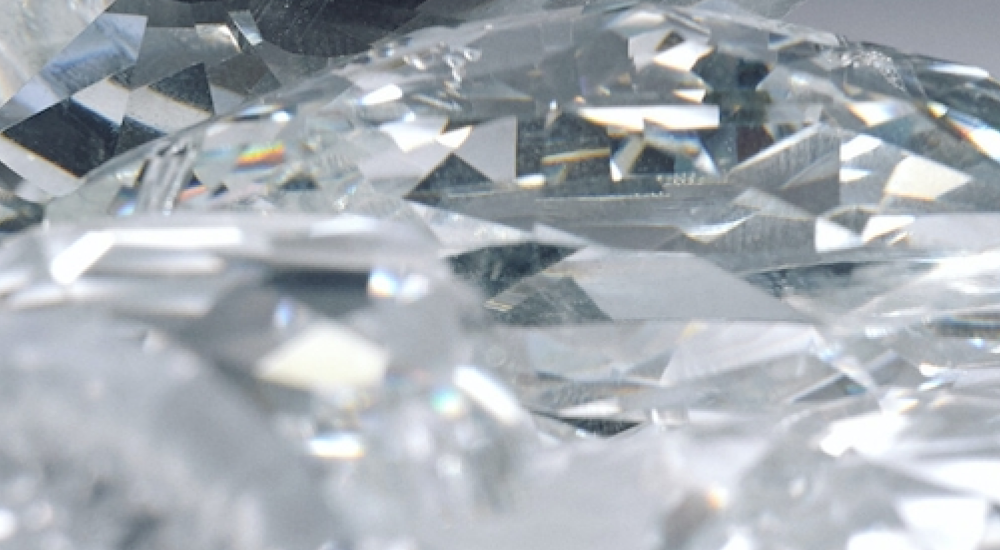
As Amazon Ads evolves, shifting towards purpose-driven measurement will set you on a trajectory for success in the age of machine learning AI. When effectively implemented, this means obtaining a more complete picture of performance through more accurate campaign measurement, which leads to more efficient strategy building and budget allocation.
Here, we outline the steps to take and explain our framework for success to help you implement purpose-driven measurement and integrate machine learning into that process in order to stay competitive in this rapidly changing environment.
Preparing for the future
Moving towards purpose-driven measurement means focusing on the complete view of performance rather than just last-touch attribution. Now is the time for advertisers to become familiar with and start utilizing tools like Amazon Marketing Cloud (AMC), which enables advertisers to achieve the ultimate goal of measuring the performance of individual campaigns.
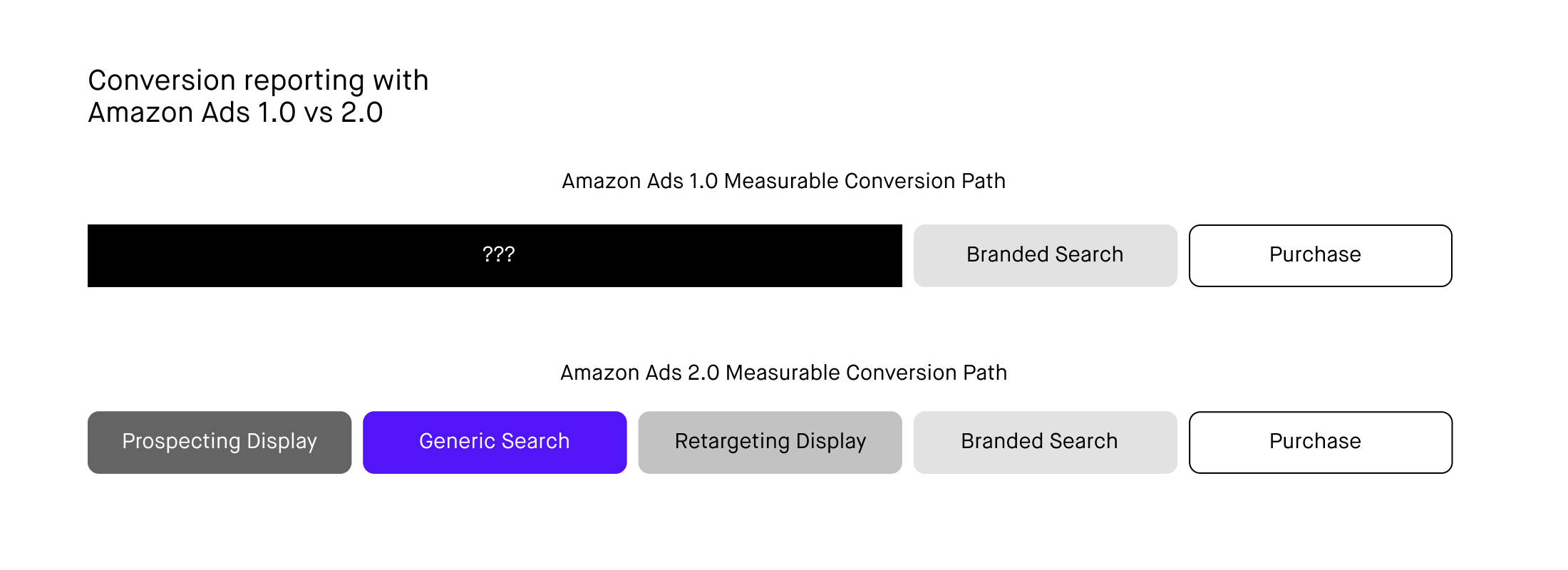
Regular search and display reports only show conversions on a last-interaction basis, meaning each purchase is attributed as a conversion to the last campaign the customer interacted with. Through the AMC, it is possible to measure customer interactions with your campaigns at all stages, rather than only the final touchpoint.
This more holistic view allows us to measure effectiveness on a purpose-driven basis.
Last interaction measurement is appropriate for low funnel campaigns such as sponsored product ads, as these are run for the purpose of driving conversion, we expect them to be the final interaction.
However, it makes little sense to measure an awareness driving campaign on the number of last interaction conversions it drives. This campaign type is not set up and optimised with this purpose in mind, your measurement should reflect that. For upper funnel campaigns, a first interaction based report would better represent the real effectiveness of these campaigns.
If you’re unsure where to begin, there are a few infallible steps to start moving in the right direction:
1. Get acquainted with Amazon Marketing Cloud (AMC), and do it NOW
Even if you’re not yet fully prepared to dive into AMC 100% (maybe you’re not yet well-versed in SQL), just start collecting the data. AMC is not retroactive, anything that happened before now is gone and cannot be retrieved later. Start with just one instance and connect your search and display entities so you don’t miss the opportunity to collect valuable data.
2. If you are already proficient in using AMC, use it to conduct a large-scale analysis
Whether you have SQL knowledge yourself, an internal team or agency that helps with more advanced SQL use, AMC can be used to perform a complete evaluation of your customer journey. Use the specific analytic capabilities to determine if your individual campaigns are doing exactly what you intend for them to do. With AMC, you can evaluate based on campaign type, target type and first vs. last interaction.
3. The takeaways from step two can inform realistic goal setting
The specific analyses achieved with AMC can help you determine what true ROAS values are for different campaign types. For example, the actual attribution available with AMC might show that your Sponsored Brands campaigns are actually involved in 50% of conversions, even though they are attributed with only 10% of reported (last interaction) conversions. You might then conclude that these campaigns achieve a ‘real’ ROAS of 5X the ROAS reporting in the ad console. This knowledge can then be used to adjust targets set for this campaign type, and may influence the budget allocation.
The key here is that AMC offers an understanding of each individual campaign performance based on what it is actually intended to do, rather than just on last-touch attribution. From here, you can start determining far more accurate targets.
Integrating machine learning AI
Machine learning tools are designed to find patterns in data much more quickly and effectively than humans, but rely on historical data, relevant performance metrics and targets to function optimally. When used in combination with purpose-driven measurement, advertisers can use them to make accurate predictions about future outcomes – something that will help improve targeting, make bidding more efficient and optimize a data-driven strategy.
Leaning into purpose-driven measurement will lead to setting realistic targets that serve as the foundation for machine learning algorithms to optimize strategies aimed towards achieving meaningful results.
When integrating machine learning tools into your advertising strategy, whether through a third-party tool or the native machine learning supported features in the ad console, it is important to keep the following in mind:
1.You need sufficient and ideally stable data
A good rule of thumb for advertising is about 60-90 days worth of data with ideally 100 conversions per month (and minimally 30). This may vary depending on what you are applying it to.
The algorithm learns from historical data to optimize bidding, so the initial targets you set should reflect recent performance. Setting targets far above past/current performance often leads to underperformance as the algorithm is forced to guess, as there is no prior data to learn from on how that performance has been achieved in the past.
The targets you set can, however, be influenced by learnings taken from AMC, where you have measured the ‘real’ ROAS vs the last interaction ROAS.
2. Slow to change, slow to judge
It is important to remember that, just like people need time to learn, so too does machine learning. Allow about 2-3 weeks for the initial “training” period at launch and be careful not to change key parameters more often than once per week. Also, allow at least seven days to observe performance changes and remain patient – we are all learning together!
Purpose-driven measurement allows you to set targets that are realistic and aligned with actual performance.
3. Machine learning is best optimized when supplemented with human knowledge
The last and most important thing to remember is that you are the expert. There are simply things that humans know and can do that machines cannot and will not ever be able to.
For example, advertisers know when their products will go on deal, something machine learning cannot predict. Therefore, the user needs to use this knowledge and adjust the tools accordingly.
Machine learning and AI are becoming a bigger part of our work than ever before, but this doesn’t mean we can just sit back and relax while the computers do all the work. Quite the opposite. Now is the time to tighten up your measurement and management protocols because what’s been working for the past five years won’t hold up in the next five.
The advancements in the measurement capabilities of Amazon Ads represents a huge step forward – the complete picture of performance can finally be made visible. Understand what kinds of data you can actually acquire and how that can help you fully leverage the machine learning AI tools that are becoming available. Work as an effective “coach,” applying machine learning and AI to the right datasets, making sure they’re complete and accurate so it never has to guess and neither do you.
Questions?
Principal Paid Advertising at DEPT®